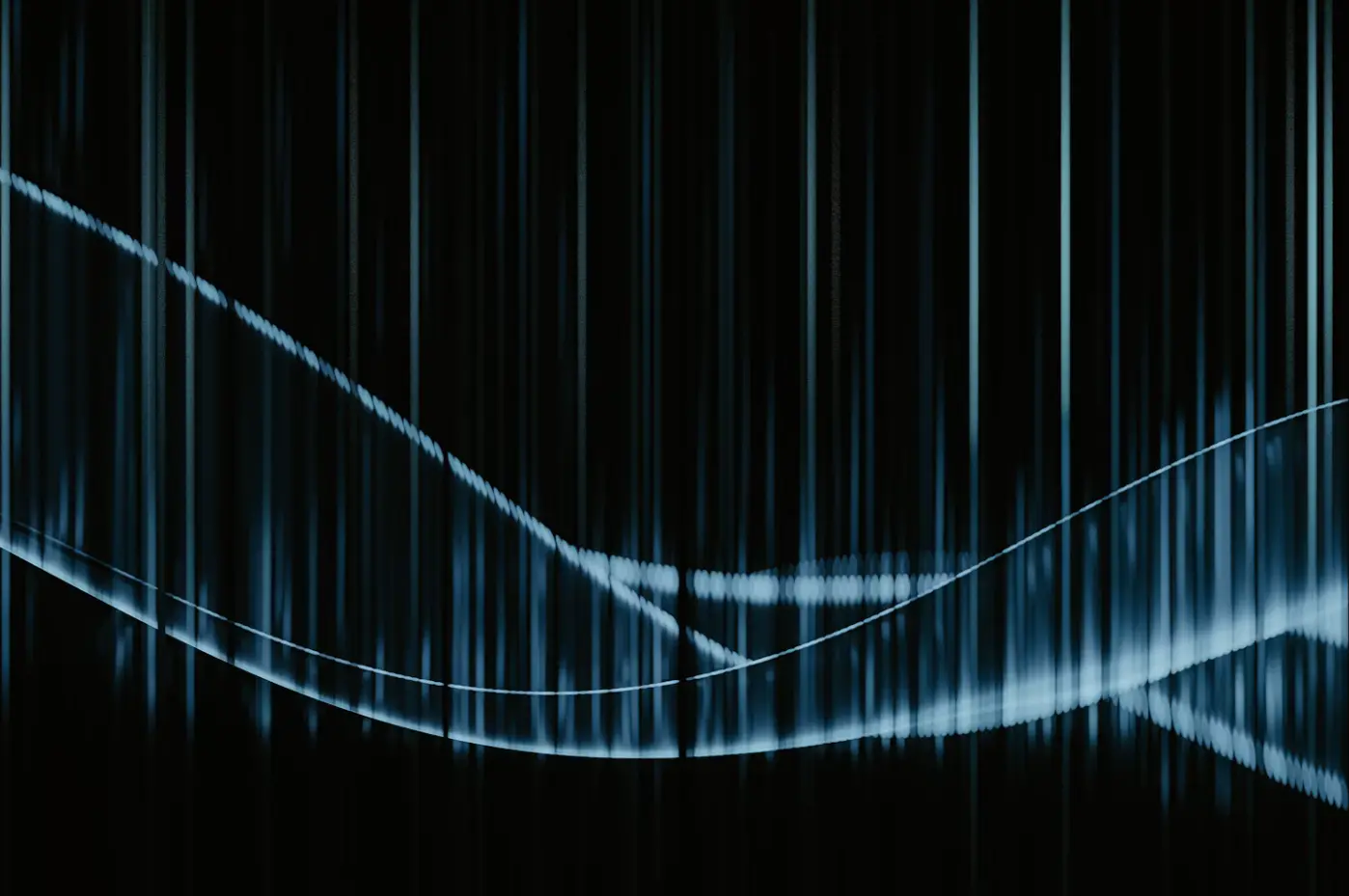
用biopython操作蛋白结构
Biopython 常用于生物序列分析,但其实它也有整个 PDB 模块,可以做简单的结构分析
import Bio.PDB as bp
本文将结构对应的实体称为 Molecule,这是我们之后看待结构文件的基本视角。Molecule 可能指单体分子、多聚体或互作的多个蛋白等
我们先来认识蛋白结构的层级关系,即 Structure > Model > Chain > Residue > Atom
Structure, Model, Chain
解析 .cif/.pdb
后,得到 Structure 对象
parser = bp.MMCIFParser()
struc = parser.get_structure('7czq', '7czq.cif')
# 获取header
struc.header
使用 struc.get_list()
获取子项。 X 射线、电镜方法解的结构只有一个 Model 对象,而核磁共振方法由于可以记录动态过程,会有多个 Model 对象
model = struc[0]
Model 的下一级是 Chain 对象,蛋白、核酸被视作 polymer Chain,糖链被视作 branched Chain
对于蛋白,Chain 在单体 Molecule 可能指不同的结构域,在多聚体 Molecule 可能指单体,在互作蛋白 Molecule 可能指参与互作的不同组分。例如,经典的抗原-抗体互作场景下,常见将抗原、抗体重链、抗体轻链分别命名为 Chain
有时,你会遇到 Molecule 是一个三聚体,每个单体上都结合抗体。于是,Model 内就有 9 个不同的 Chain,即 3 个拷贝的抗原单链、抗体重链、抗体轻链
# 列出所有Chain
chains = model.get_list()
# 选择Chain H
chain = model['H']
# 显示Chain id
chain.id
有时,pdb 的 3D view 中仅显示干净的单个 Molecule,但下载的 .pdb/.cif
在 pyMol 中显示多个 Molecule(如pdbid: 4jtv)。biopython 读取的结构会与 pyMol 一致,仍是单个 Model,内部有多个 Molecule 对应的 Chain
Residue, Atom
使用 chain.get_list()
可得当前 Chain 的 Residue 列表。resseq
代表 Residue site id,与 pdb 上 auth index 相同
[<Residue ALA het= resseq=27 icode= >,
<Residue TYR het= resseq=28 icode= >, ...]
与 Chain 有直接相互作用的小分子,也会被纳入当前 Chain 作为 Residue,这种情况用 het
表示。例如 W
代表水,H_NAG
代表N-乙酰葡糖胺,可查询化学成分字典
<Residue NAG het=H_NAG resseq=1 icode= >
<Residue HOH het=W resseq=904 icode= >
最后,icode(insertion code)是为了处理 insertion
# Residue三字母简写
res.resname
# 编号,格式为(hetero, resid, insertion_code)
res.id
同理,从 Residue 可以 res.get_list()
获得 Atom 列表,其中 CA, CB
分别代表 alpha C 和 Beta C,可用 atom = res['CA']
选取 alpha C
# 编号
atom.id
# 坐标
atom.coord
atom1 - atom2
可以计算原子距离,这允许我们计算残基间的相互作用
结构字典
除了解析为 Structure 外,.cif/.pdb
数据可以被转为结构字典
struc_dict = bp.MMCIF2Dict.MMCIF2Dict('7czq.cif')
字典的全部分组可参考 Category Group Index mmcif,这里介绍最常用的。pdb 格式规范并不要求每个分组、属性都存在
struct 分组,展示结构的基本信息
pd.DataFrame({
'pdbid': struc_dict['_struct.entry_id'],
'title': struc_dict['_struct.title'],
'des': struc_dict['_struct.pdbx_descriptor'],
})
entity 分组,展示了所有 entity,内容同 pdb Web Structure viewer
pd.DataFrame({
'id': struc_dict['_entity.id'],
'type': struc_dict['_entity.type'],
'des': struc_dict['_entity.pdbx_description'],
'count': struc_dict['_entity.pdbx_number_of_molecules'],
'weight': struc_dict['_entity.formula_weight'],
})
entity_poly 分组,仅展示 polymer,有 chain id、规范氨基酸序列等信息。注意 pdbx_seq_one_letter_code
属性也是氨基酸序列,但使用 (UNK)
而不是 X
记录未知氨基酸,使用 (XXX)
记录了除氨基酸以外的分子。更多讨论参见这里
两种氨基酸序列都包含未存储在 Structure 但被作者手动加入的氨基酸,也就是下图的灰色部分。因此比遍历取出每个 Residue 更合理
pd.DataFrame({
'id': struc_dict['_entity_poly.entity_id'],
'type': struc_dict['_entity_poly.type'],
'chain': struc_dict['_entity_poly.pdbx_strand_id'],
'seq': struc_dict['_entity_poly.pdbx_seq_one_letter_code_can'],
})
pdbx_nonpoly_scheme 分组,展示因与某 Chain 有互作,被分配到 Chain 下的小分子。其中 asym_id
代表该小分子的唯一 id
pd.DataFrame({
'id': struc_dict['_pdbx_nonpoly_scheme.entity_id'],
'name': struc_dict['_pdbx_nonpoly_scheme.mon_id'],
'chain': struc_dict['_pdbx_nonpoly_scheme.pdb_strand_id'],
'site': struc_dict['_pdbx_nonpoly_scheme.auth_seq_num'],
'asym_id': struc_dict['_pdbx_nonpoly_scheme.asym_id'],
})
struct_conn 分组,展示链间的相互作用
pd.DataFrame({
'id': struc_dict['_struct_conn.id'],
'type': struc_dict['_struct_conn.conn_type_id'],
'chain_x': struc_dict['_struct_conn.ptnr1_label_asym_id'],
'chain_y': struc_dict['_struct_conn.ptnr2_label_asym_id'],
'site_x': struc_dict['_struct_conn.ptnr1_label_seq_id'],
'site_y': struc_dict['_struct_conn.ptnr2_label_seq_id'],
'residue_x': struc_dict['_struct_conn.ptnr1_label_comp_id'],
'residue_y': struc_dict['_struct_conn.ptnr2_label_comp_id'],
'atom_x': struc_dict['_struct_conn.ptnr1_label_atom_id'],
'atom_y': struc_dict['_struct_conn.ptnr2_label_atom_id'],
'dist': struc_dict['_struct_conn.pdbx_dist_value'],
})
citation 分组展示引用信息
pd.DataFrame({
'title': struc_dict['_citation.title'],
'journal': struc_dict['_citation.journal_abbrev'],
'year': struc_dict['_citation.year'],
'doi': struc_dict['_citation.pdbx_database_id_DOI'],
})
输出
使用 io 模块输出结构文件
# 输出pdb
io = bp.PDBIO()
io.set_structure(struc)
io.save('t.pdb')
# 输出cif
io = bp.MMCIFIO()
io.set_structure(struc)
io.save('t.cif')
biopython 不支持 entity 的删除,只能通过新建对象达成删除目的
# 保存某些链
class ChainSelect(bp.Select):
def accept_chain(self, chain):
if chain.id in ['A', 'B']: return True
else: return False
io = bp.MMCIFIO()
io.set_structure(struc)
io.save('t.cif', ChainSelect())
# 将各链分别保存
for chain in struc.get_chains():
io.set_structure(chain)
io.save(struc.get_id() + "_" + chain.get_id() + '.cif')
去除水、小分子等异质 Residue,也需要新建对象
from Bio.PDB.Residue import Residue
from Bio.PDB.Atom import Atom
from Bio.PDB.Chain import Chain
from Bio.PDB.Model import Model
from Bio.PDB.Structure import Structure
new_struct = Structure('new')
for model in struct:
tmodel = Model(model.id)
for chain in model:
tchain = Chain(chain.id)
for res in chain:
if res.id[0].strip() == '':
tchain.add(res)
tmodel.add(tchain)
new_struct.add(tmodel)
相对溶剂可及性(rSASA)
biopython 只支持 SASA 的计算,要获取 rSASA 还需手动除以每种氨基酸的最大 SASA。由于最大 SASA 的认定不同,计算结果可能和其他软件有差异,但趋势是一致的
from Bio.PDB.SASA import ShrakeRupley
sr = ShrakeRupley()
sr.compute(new_struct)
max_sasa = {
'ALA': 121, 'ARG': 265, 'ASN': 187, 'ASP': 187,
'CYS': 148, 'GLU': 214, 'GLN': 214, 'GLY': 97,
'HIS': 216, 'ILE': 195, 'LEU': 191, 'LYS': 230,
'MET': 203, 'PHE': 228, 'PRO': 154, 'SER': 143,
'THR': 163, 'TRP': 264, 'TYR': 255, 'VAL': 165
}
rsasa_dict = {'site': [], 'residue': [], 'rsasa': []}
for res in new_struct[0]['A']:
rsasa = round(res.sasa / max_sasa[res.resname], 2)
rsasa_dict['site'].append(res.id[1])
rsasa_dict['residue'].append(res.resname)
rsasa_dict['rsasa'].append(rsasa)
rsasa_df = pd.DataFrame(rsasa_dict)
rsasa_df.loc[rsasa_df.rsasa > 1, 'rsasa'] = 1
sr.compute()
如果传入 Structure 对象,会在全局视野计算,Chain 之间的互作也会影响 SASA。如果传入 Chain 对象,SASA 就不受 Chain 之间互作影响
结语
至此,简单的三维结构分析就介绍完毕。玩的开心